Can you run a social media account only using AI?
I recently took some time off work and thought I’d use the opportunity to answer this question.
See, it’s hard coming up with new content consistently, but I’m a bit of a purist when it comes to what I share on social media and how I share it. We’ve even covered this before while addressing how the Buffer Content team uses AI in our creative process.
But I thought, for science, I’d do this experiment and answer one of the AI questions that creators might be deeply curious about: how does AI content on LinkedIn perform?
What I did and the experiment parameters
I focused on a weeklong period, scheduling all content through Buffer. This approach allowed me to analyze the performance of AI-generated content, which I did using the stats LinkedIn makes available.
For this experiment, I focused my efforts exclusively on LinkedIn, where I’ve been building my personal brand, over a one-week period from November 6th to November 12th, 2023, and I used LinkedIn’s platform analytics to track and compile the performance data of the AI-written content.
To generate the posts, I used three leading AI tools to not seem biased – Buffer’s AI Assistant, Claude AI, and ChatGPT. Also, these tools specifically gave me the best chance of creating content to a certain level of quality I hoped for.
To keep the experiment parameters strict, 100 percent of the content was AI-generated based on various prompts. The only exception was some light proofreading before publishing.
I assigned each one the task of drafting content for a set number of days:
Buffer’s AI Assistant – three days of LinkedIn posts Claude AI – two days of LinkedIn posts ChatGPT – two days of LinkedIn posts
While I tried to make sure each tool created a set amount of content for the days I was assigned, I used a mix of all the tools to refine the content. So, there’s no one tool I’d say is better, but one common thread across all the tools was that the more context I offered, the better the content I got.
In terms of prompts, I supplied the AI tools with:
Original prompts I crafted specifically for my target audience and messaging goals. An existing high-performing content prompt shared by Mike Cardona’s 90-Day Content Library prompt. Prompts I had previously created for Buffer’s Assistant (these are usually featured in our Social Media newsletter).
I made sure all the prompts reflected my content pillars of personal brand building, career growth and AI, so I could stay on brand.
While there was no true scientific or data-driven basis for this experiment (I truly just wanted to see if my audience would notice), here are some of the boundaries I set for myself:
All content has to be totally AI-generated – only proofreading can be done manuallyI can tweak prompts infinitely to get better results, but nothing moreI can hop in to engage with commentsAll content is scheduled in Buffer (*wink wink*) and sorted with our new Tags feature.
These boundaries helped me force myself away from perfectionism, which allowed me to save time and work quickly. But it also limited the creativity and personal perspective I could put into the content, a major limitation of AI-only content.
The goal with these controlled parameters was to test how an audience would respond if they received a week of content from my account written entirely by AI, with minimal human oversight. Here are the results.
The results
Now, I need to share one thing about me: my data analysis skills aren’t the strongest. So I’ve had to turn to AI at this stage of the experiment as well.
Upfront analysis of content performance
Here are the results of the AI-generated content from November 6th to 12th, 2023.
Total impressions for that week: 9,624Average daily impressions: 1,375Total engagements for that week: 151 Average daily engagements: 22
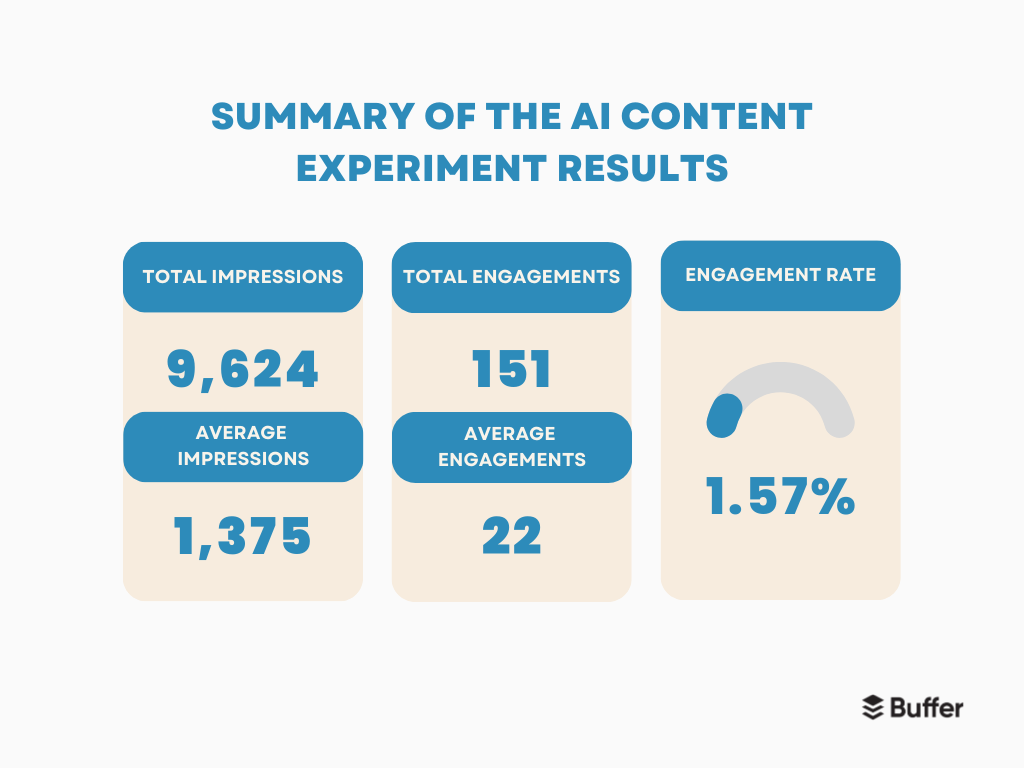
Overall, while the engagement rates could potentially be higher, the AI-written posts fared well, and all my objections are more about the quality of the content.
The impressions and total engagement numbers indicate an engaged audience for content written automatically with minimal oversight. Monitoring this over a longer period could provide insight into real performance trends. But for the week I focused on, the posts achieved solid metrics.
Now, let’s dive deeper into the data.
Impressions and engagements
Over the 7-day experiment, the AI-generated content garnered significant visibility totaling 9,624 impressions, generating 151 user interactions through likes, comments, and shares registered as engagements.
On an average daily basis, this broke down to:
1,375 impressions 22 engagements
Compared to my overall LinkedIn averages, this week massively over-indexed for visibility and response:
Average impressions for Nov 6-12 are about 11% higher than a typical week over the previous 3-month period.
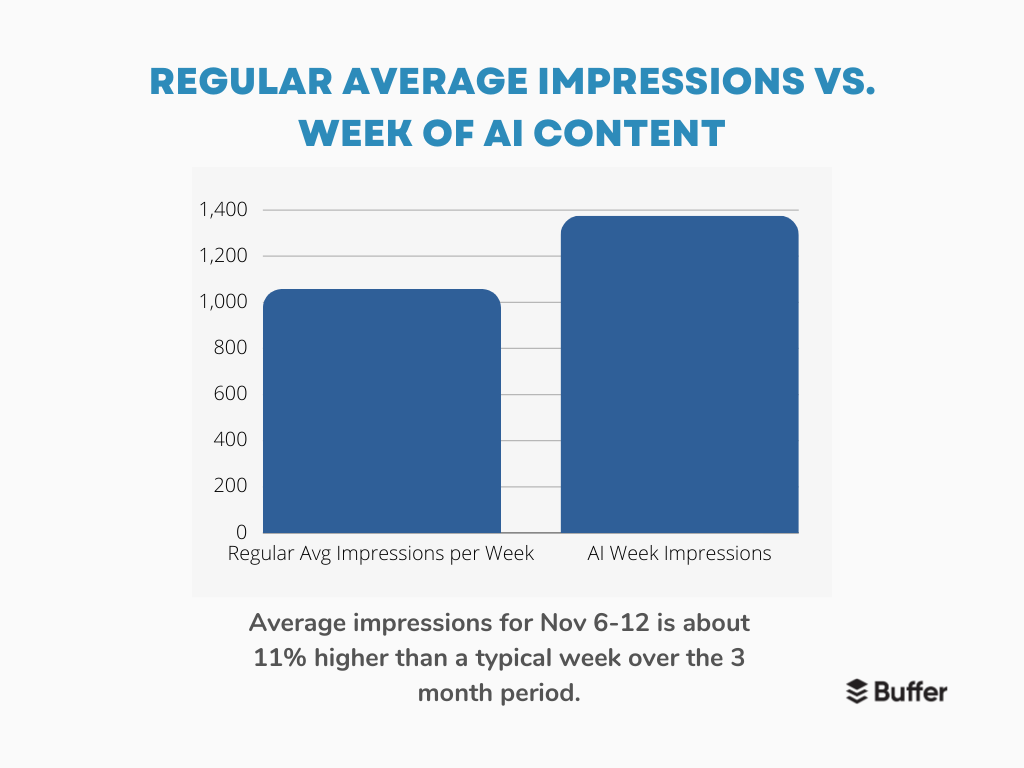
Average engagements for Nov 6-12 are over 75% higher than a typical week.
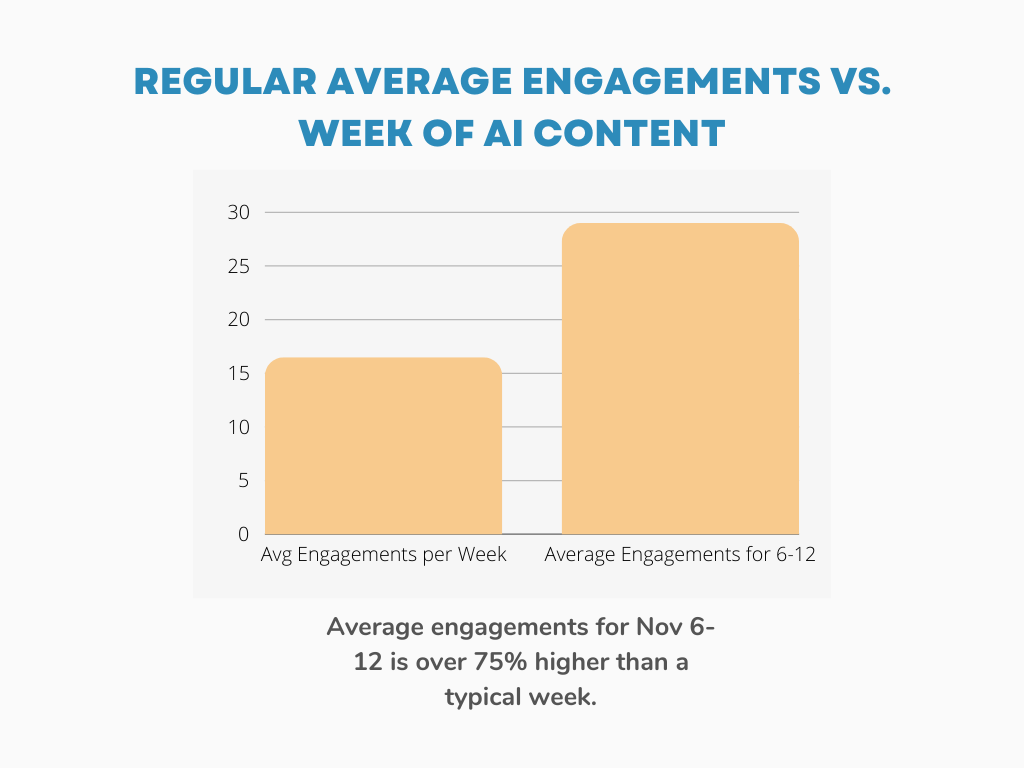
Based on this, we can assume that the AI-generated content resonated in terms of sheer reach and raw interactions generated based on elevated volumes from historical baselines.
At an aggregate weekly level, achieving nearly 10,000 impressions demonstrates a meaningful scale of discovery. And while I wish engagement was higher (don’t we all), crossing 150 actions or nearly 25 per day is a strong baseline response indicating the AI-produced posts intrigued my audience.
Engagement rates
We can also examine user behavior through the engagement rates, also known as the ratio of interactions to impressions.
Over the seven-day stretch, the posts achieved an average 1.57 percent engagement rate, which is taken from the 151 total engagements generated divided by the 9,624 aggregate impressions.
Breaking down daily engagement rate provides additional context:
Best Performing Day: November 6th at 3.5 percent rate Worst Performing Day: November 12th at 1 percent rateRemaining days ranged between one to three percent
The best performing day was a Monday, and the worst was a Sunday, so the downward trend isn’t worrying and matches with expectations of LinkedIn content performance.
From this analysis, I can tell that scheduling posts earlier in the week could be better for engagement.
Actual content performance
Now, moving on from the averages and aggregates of the whole week, one major note from the performance of content across the week is that actionable advice directly helping readers succeed at something performed dramatically better.
Digging deeper, the highest traction post from November 6th covering actionable online writing tips saw 60 user interactions measured against 1,699 impressions for a 3.5 percent engagement rate.
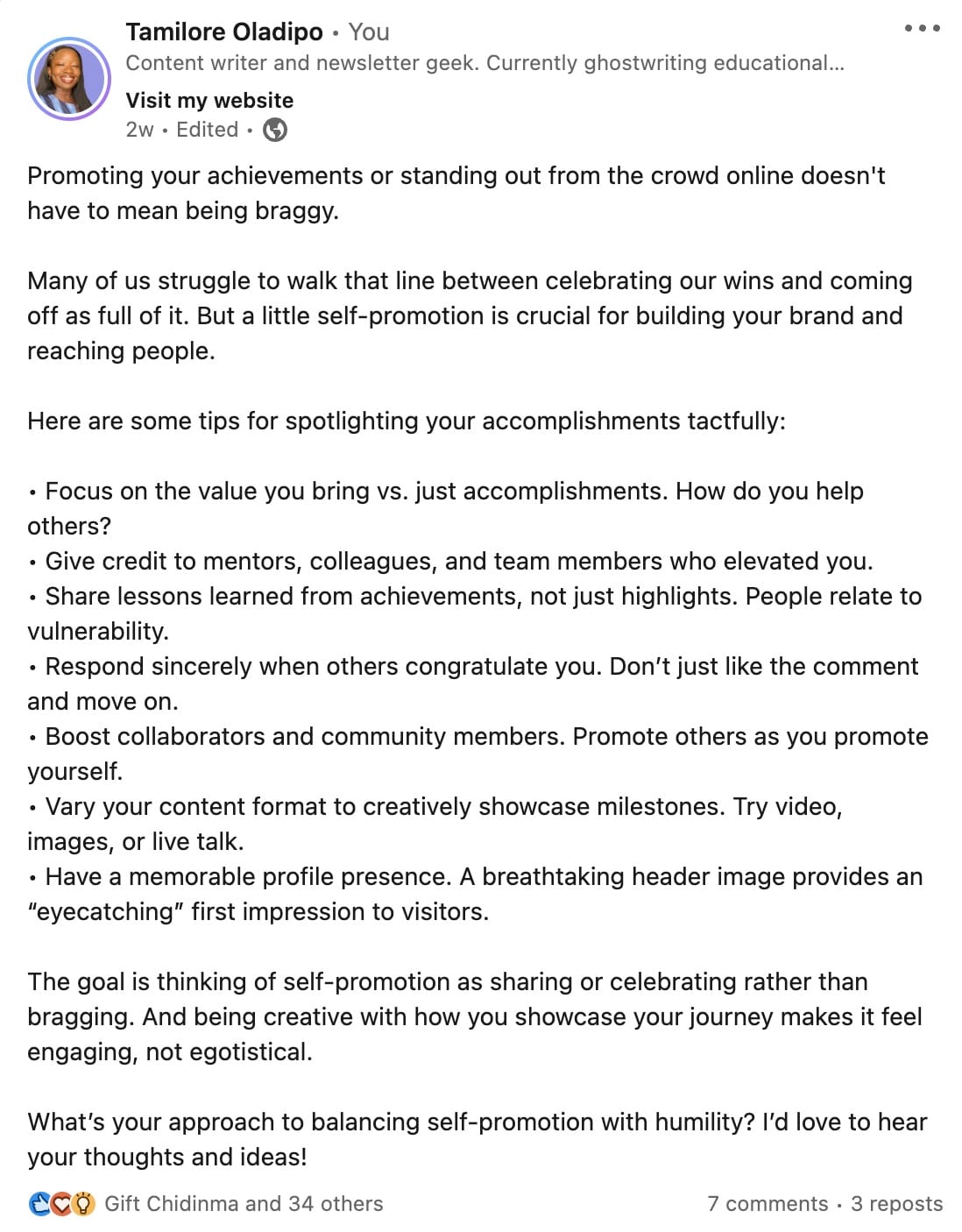
Comparatively, November 12th’s lower-performing post was more conceptual/philosophical as an overview of AI branding basics and saw only 10 engagements from 967 views – a one percent rate.
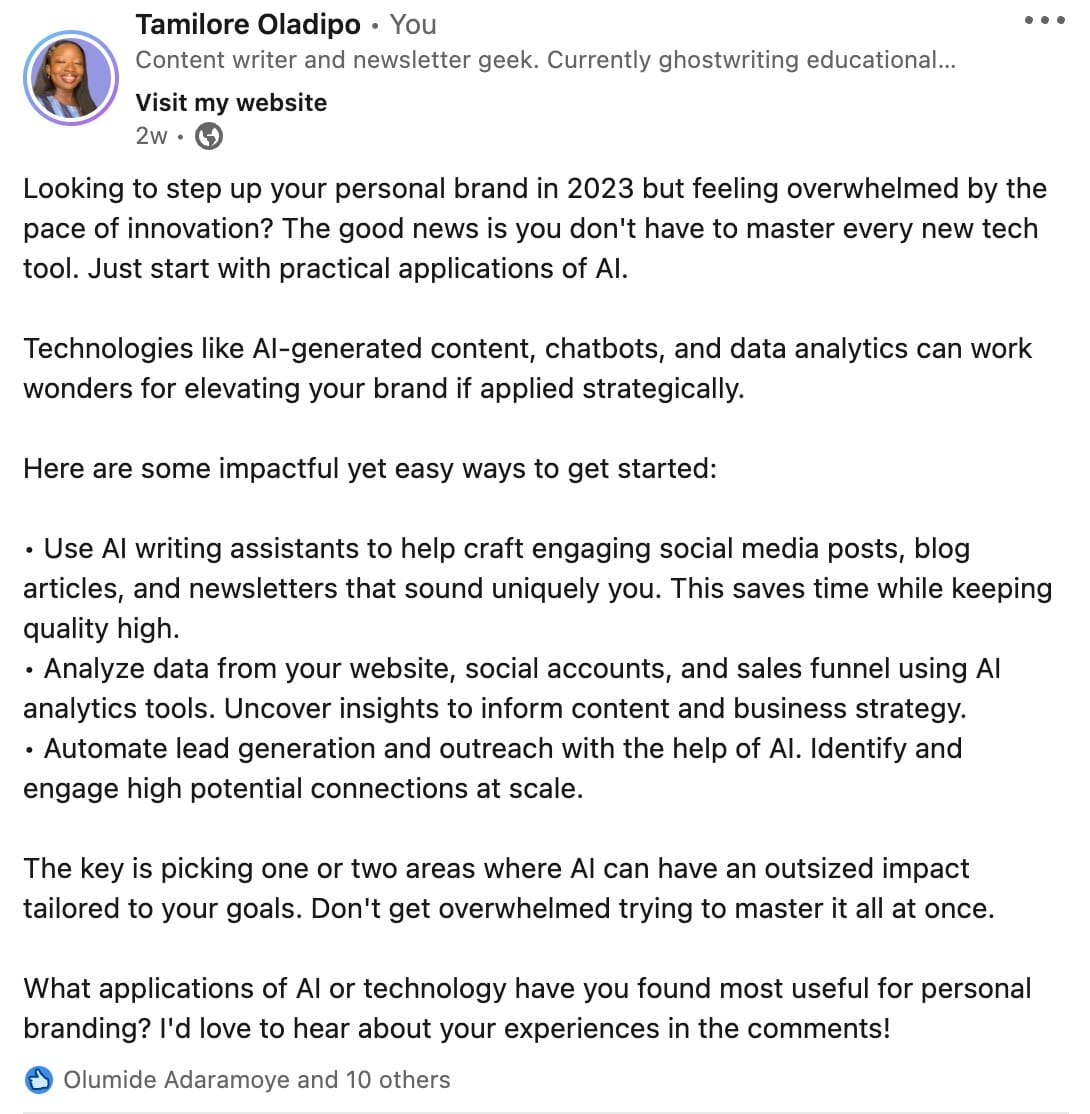
Analyzing the most and least engaging pieces by topic reveals that my audience appears to strongly prefer immediately applicable “how-to” improvements. Despite its informational value, forward-looking thought leadership often overwhelms or loses portions of audiences.
This trend recurred throughout the week, with practical skill-building content significantly outperforming sophisticated but more passive consumption pieces.
The clear takeaway centers on bite-sized, tactical content better commanding audience investment – aligning rationally with their immediate growth needs.
Time Series Analysis
I got ChatGPT to make a chart plotting daily impressions throughout the week.
Some key observations and takeaways from this analysis:
Peak days: There was a significant uptick in the engagement at the start of the week, with Mondays and Tuesdays showing the highest levels of interaction.Mid-week trends: A noticeable dip occurred mid-week, particularly on Wednesday and Thursday, indicating less audience activity during these days.Weekend insights: Despite a general perception of weekends being less favorable for engagement, our Saturday posts performed relatively well, although a drop was observed on Sunday.
What went well
So, let’s talk about the good stuff from this AI content experiment. When I dove into the numbers and the ups and downs of the week, a few cool things really stood out.
First off, the AI was running the show here with just a hint of a human touch through prompts and context sharing. This gave me a fresh look at how content lands without the added personal perspective. And surprise, surprise, it turns out that AI can churn out stuff that not only grabs attention but also gets people talking and engaging. Pretty cool.
When we stack this week’s numbers against previous ones, it’s clear that AI isn’t just a one-hit wonder as a creative assistant. We’re talking consistent impact, pulling in views and interactions beyond what we usually see. However, this isn’t just random luck but a combination of a few things:
The trust I’ve built with my original content played a big role in the performance of the AI content. I typically don’t publish every day of the week, but when I do, I get engagement. That’s a result of trust built over time with my audience. My advice: focus on building that trust. A deep understanding of what’s likely to resonate with my audience through content pillars. I didn’t just select the random ideas I got from the AI tools, I made sure to refine the content till it matched what I knew people would expect from me.
Now, let’s talk topics. The most popular post offered practical advice focused directly on the reader – how to improve their writing skills as a creator. The least popular took a different, broader angle discussing AI applications for personal branding, ending up more conceptual and abstract for the average reader. Some key takeaways:
Posts providing tangible tips, tricks or advice for readers scored much higher engagement than big-picture think piecesActionable content helping users make progress resonated more than thought leadership-style ideas Practicality over philosophy when aiming to drive interactions
This suggests focusing content on bite-sized, practical takeaways readers can immediately apply will reliably yield higher engagement. Whereas more conceptual or forward-looking themes may lose or overwhelm some users despite being intellectually interesting.
What didn’t go well
Prompting AI tools is more an art than a science, which means there’s no precise way to get it to truly “sound human” unless you interfere and edit the content it generates.
For example, when I would share a prompt, the first answer would almost always be extremely flawed. Some common mistakes were repetition and unnecessary lists. AI tools also have a weird habit of capitalizing in weird places – and I don’t write like that. I could always share additional prompts to get the results closer to sounding like me, but it wasn’t perfect.
Conclusion
So, yes, I published AI-generated content for a week straight, and no one noticed. In fact, my engagement stayed the same and was even better in some cases.
My next move is all about fine-tuning. Here are some next steps I’d take away from this experiment:
My content pillars work best when they follow the actionable advice route, so I’ll prioritize that content on LinkedIn from now on.Long-form content is a winner – all the posts were over 350 words, and the performance wasn’t hurt by length.This is more of a personal thing, but I’ll always tweak the AI voice and style to match mine. It was uncomfortable to notice things I would have removed/changed if I hadn’t set such structure parameters.
If you’re like me and have built up trust with your audience, struggle with consistency, or just want more ways to frame your ideas, letting AI take a swing at expanding your reach seems like a no-brainer.